欧州の研究者をお招きして「第16回マテリアル探索自動化・自律化人材育成セミナー」を開催しました
- 形式:ハイブリッド
オンライン参加ご希望の方はその旨をGoogle formにご記入ください。
後日、ZOOMのURLを直接お知らせします。
- 言語:英語
- お申込:人数把握のためできる限り参加登録をお願いいたします。
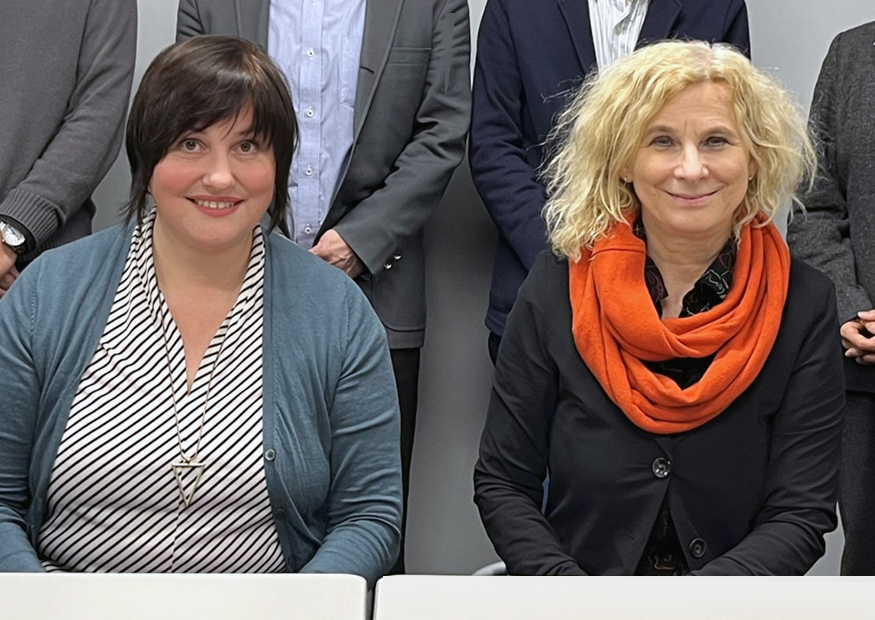
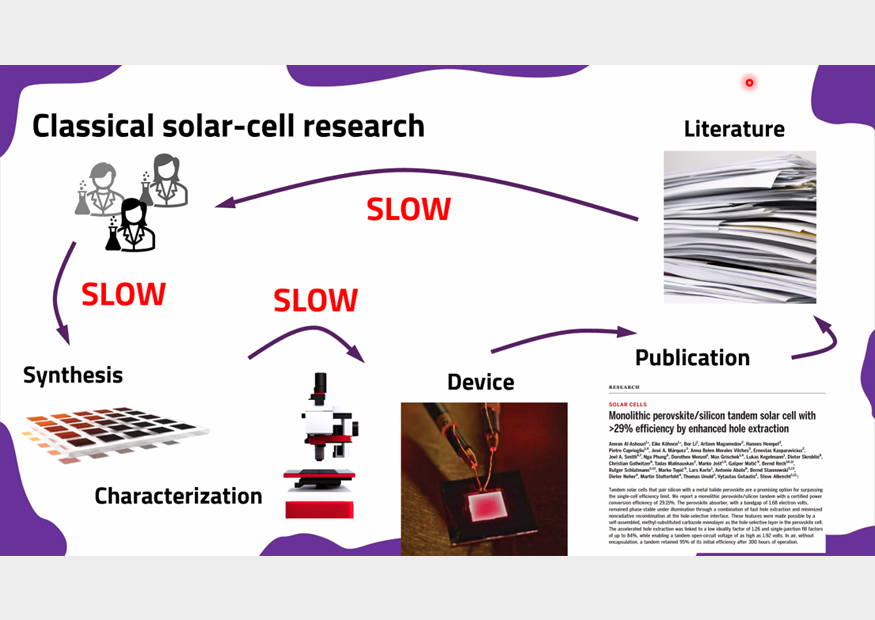
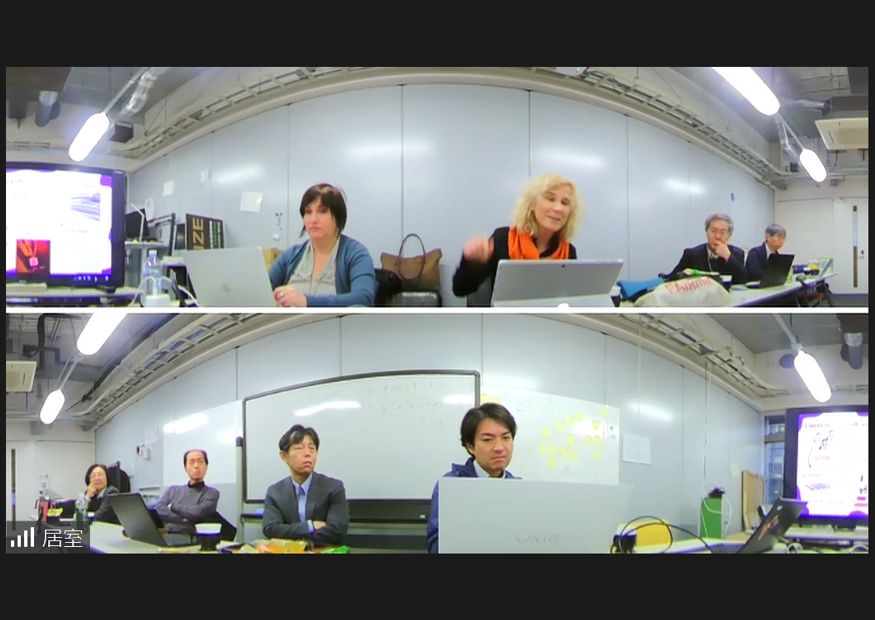
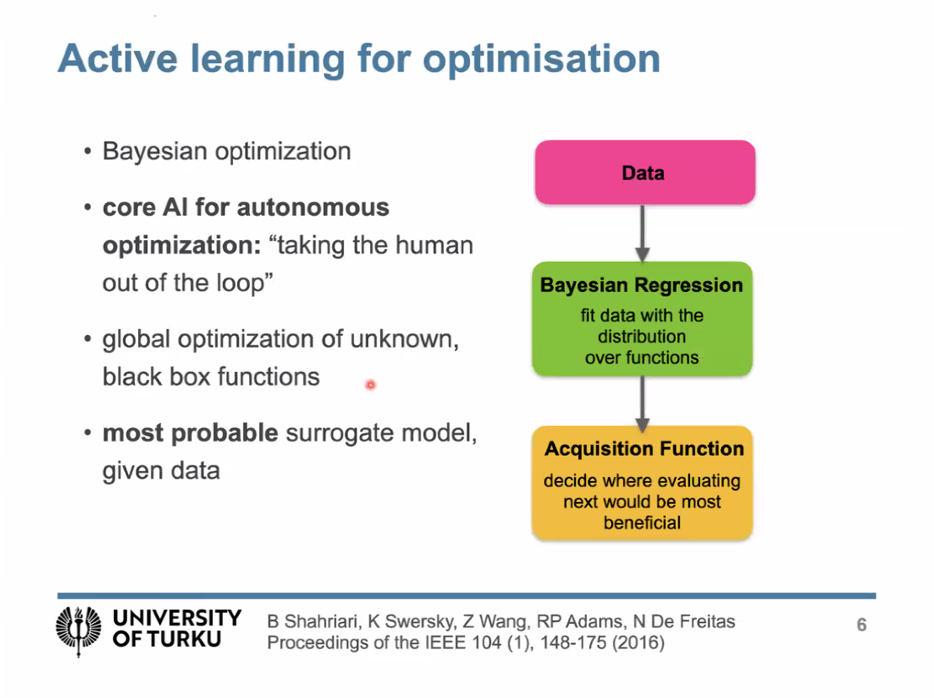
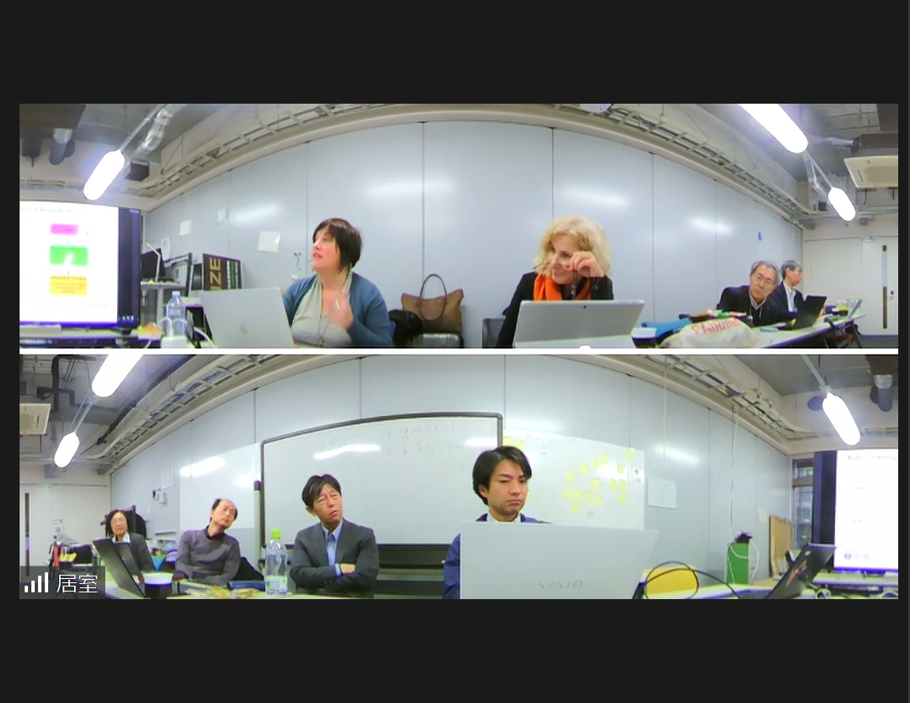
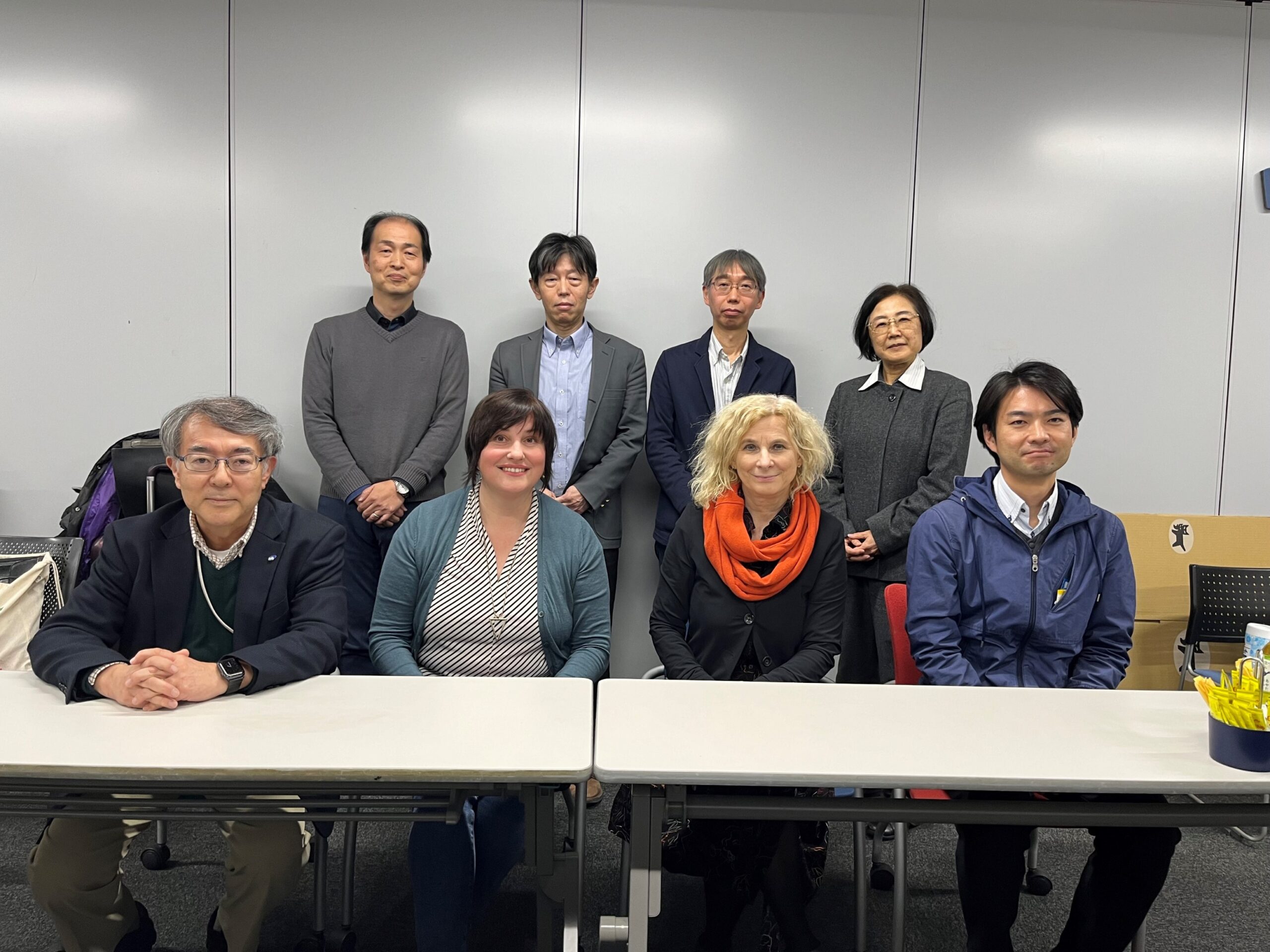
解説:21世紀、材料科学の進化はデータ駆動の時代を迎えています。このセミナーシリーズで今回は欧州のClaudia Draxl教授とMilica Todorović准教授をお呼びして,日々蓄積される膨大なデータを知識と価値に変えるための戦略やテクニックを紹介して頂きました。FAIRデータ基盤の重要性や異質なデータを活用した材料科学AI技術が紹介されました。大学院生から大学教授、産業界の研究者まで、材料科学でのデータからの知識獲得やAIに興味を持つすべての方を対象として開催されました。(NIMS木野日織)
Jacobsson, T.J., Hultqvist, A., García-Fernández, A. et al. An open-access database and analysis tool for perovskite solar cells based on the FAIR data principles. Nat Energy 7, 107–115 (2022).
Milica先生からはベイズ最適化を用いた分子構造の探索等、MEEPの自律探索と強く関係する話題をいただき、MEEPのPOC1:自律実験・自律計測・計算科学を用いたマテリアル探索空間拡張の参考になりました。
ACS sustainable Chem Eng. 10, 9469 (2022)
NOMAD
NOMAD is a data infrastructure service and software that let's you manage, share, and publish materials science research data.
開催要項
日時: 2023年12月19日 (火) 14:00-16:00
- 形式:ハイブリッド
オンライン参加ご希望の方はその旨をGoogle formにご記入ください。
後日、ZOOMのURLを直接お知らせします。
- 言語:英語
- お申込:人数把握のためできる限り参加登録をお願いいたします。
(Google form 申し込み終了)
References
[1] https://fairmat-nfdi.eu
[2] M. Scheffler, M. Aeschlimann, M. Albrecht, T. Bereau, H.-J. Bungartz, C. Felser, M. Greiner, A. Groß, C. Koch, K. Kremer, W. E. Nagel, M. Scheidgen, C. Wöll, and C. Draxl, Nature 604, 635 (2022).
[3] M. Kuban, S. Gabaj, W. Aggoune, C. Vona, S. Rigamonti, and C. Draxl, MRS Bulletin 47, 991 (2022).
[4] T. Bechtel, D. Speckhard, J. Godwin, and C. Draxl, preprint.
[1] npj Comput. Mater., 5, 35 (2019)
[2] Beilstein J. Nanotechnol. 11, 1577-1589 (2020), Adv. Func. Mater., 31, 2010853 (2021)
[3] Adv. Sci. 7, 2000992 (2020)
[4] ACS Appl. Mater. Interfaces 14 (10), 12758-12765 (2022)
[5] J. Chem. Theory Comput. 17, 1955 (2020)
[6] MRS Bulletin 47, 29-37 (2022)
[7] ACS Sustainable Chem. Eng. 10, 9469 (2022)
- カテゴリー
- Seminar